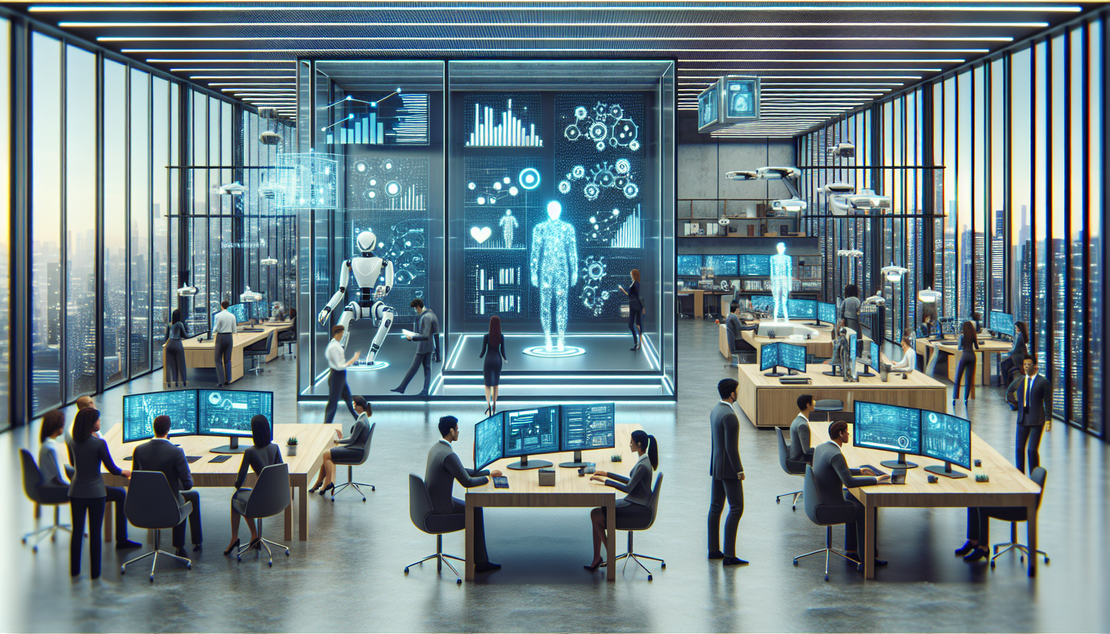
The Power of Machine Learning in Business Automation
- Rajdeep Barad
- Machine learning , Business automation , Ai applications , Predictive analytics
- February 2, 2025
The Power of Machine Learning in Business Automation
The Benefits of Machine Learning in Business Automation
Machine learning has revolutionized the way businesses operate by automating processes and providing valuable insights. Here are some key benefits of integrating machine learning into business automation:
Improved Decision-Making Accuracy: Machine learning algorithms can analyze vast amounts of data to make data-driven decisions more accurately than humans.
Pro Tip: Ensure your machine learning models are regularly updated with fresh data to maintain accuracy over time.
Increased Efficiency and Productivity: Automation of repetitive tasks saves time and allows employees to focus on more strategic initiatives.
Pro Tip: Identify key processes that can be automated to streamline operations and boost productivity.
Cost Savings Through Automation: By automating manual processes, businesses can reduce labor costs and minimize errors, leading to significant cost savings.
Pro Tip: Conduct a cost-benefit analysis to identify areas where automation can provide the highest return on investment.
Personalization and Enhanced Customer Experience: Machine learning enables personalized recommendations and targeted marketing campaigns, enhancing the overall customer experience.
Pro Tip: Use machine learning algorithms to analyze customer data and tailor products or services to meet specific needs.
Scalability and Flexibility in Operations: Machine learning systems can scale to handle large volumes of data and adapt to changing business requirements seamlessly.
Pro Tip: Invest in cloud-based machine learning platforms for greater scalability and flexibility in operations.
Applications of Machine Learning in Business
Machine learning finds diverse applications in business automation, driving innovation and efficiency. Here are some common applications:
Predictive Analytics for Business Forecasting: Machine learning models can analyze historical data to make accurate predictions about future trends and outcomes.
Pro Tip: Use time series forecasting techniques in machine learning to predict future business metrics with precision.
Customer Segmentation and Targeting: Machine learning algorithms can segment customers based on behavior and preferences, enabling targeted marketing strategies.
Pro Tip: Implement clustering algorithms to group customers with similar attributes for more effective targeting.
Chatbots and Customer Service Automation: AI-powered chatbots can handle customer queries and provide support round the clock, improving customer service efficiency.
Pro Tip: Train chatbots with natural language processing models to enhance conversation flow and customer satisfaction.
Fraud Detection and Cybersecurity: Machine learning can detect anomalies in data patterns to prevent fraudulent activities and enhance cybersecurity measures.
Pro Tip: Implement a combination of supervised and unsupervised learning techniques for robust fraud detection systems.
Supply Chain Optimization: Machine learning algorithms can optimize supply chain operations by forecasting demand, improving inventory management, and enhancing logistics.
Pro Tip: Integrate machine learning models with IoT sensors for real-time data analytics and smart decision-making in the supply chain.
Implementing Machine Learning in Business
Successfully implementing machine learning in business requires a strategic approach and collaboration between data scientists and business stakeholders. Here are key steps in the implementation process:
Data Collection and Preprocessing: Collect relevant data from various sources and preprocess it to ensure consistency and accuracy for model training.
Pro Tip: Use data cleaning techniques like handling missing values and outliers to improve the quality of your dataset.
Model Selection and Training: Select the appropriate machine learning model based on the business problem and train it using labeled data to make accurate predictions.
Pro Tip: Experiment with different models and hyperparameters to optimize performance and achieve the desired outcomes.
Integration with Existing Systems: Integrate machine learning models with existing business systems and workflows to automate processes and deliver insights seamlessly.
Pro Tip: Leverage APIs for smooth integration of machine learning models with popular business applications and platforms.
Monitoring and Refining the Models: Continuously monitor the performance of machine learning models, collect feedback, and refine the models to adapt to changing business dynamics.
Pro Tip: Implement A/B testing to compare the performance of the existing model with the updated version and make data-driven decisions for model improvements.
Collaboration Between Data Scientists and Business Stakeholders: Foster collaboration between data science teams and business stakeholders to align machine learning initiatives with business goals and objectives.
Pro Tip: Organize regular meetings and communication channels to ensure transparency and shared understanding between technical and non-technical teams.
Challenges and Considerations in Adopting Machine Learning for Business Automation
While the benefits of machine learning for business automation are substantial, several challenges and considerations need to be addressed for successful adoption. Here are some key challenges:
Data Security and Privacy Concerns: Safeguarding sensitive data and ensuring compliance with data privacy regulations are critical considerations when implementing machine learning solutions.
Pro Tip: Implement encryption techniques and access controls to protect data privacy and prevent unauthorized access.
Availability of Quality Data: Data quality and quantity play a crucial role in the success of machine learning projects. Ensuring clean, relevant data is essential for training accurate models.
Pro Tip: Use data validation techniques and data augmentation methods to enhance the quality and quantity of training data for machine learning models.
Skill Gap in Machine Learning Expertise: A shortage of skilled data scientists and AI experts can hinder the implementation of machine learning projects in organizations.
Pro Tip: Invest in training programs and upskilling initiatives to build an in-house team of data scientists and machine learning specialists for long-term success.
Integration with Legacy Systems: Integrating machine learning solutions with legacy systems and infrastructure can pose compatibility and scalability challenges for businesses.
Pro Tip: Adopt a phased approach to system integration, starting with pilot projects to assess compatibility and gradually scale up implementation across the organization.
Ethical Implications of Automated Decision-Making: Ensuring transparency, accountability, and fairness in automated decision-making processes is crucial to address ethical concerns and maintain trust with stakeholders.
Pro Tip: Establish clear governance frameworks and ethical guidelines for machine learning algorithms to promote responsible and ethical AI practices within the organization.
Ready to transform your digital vision?
Get in touch with us to explore how our cutting-edge solutions can elevate your business to new heights. Contact us today!
Get in Touch