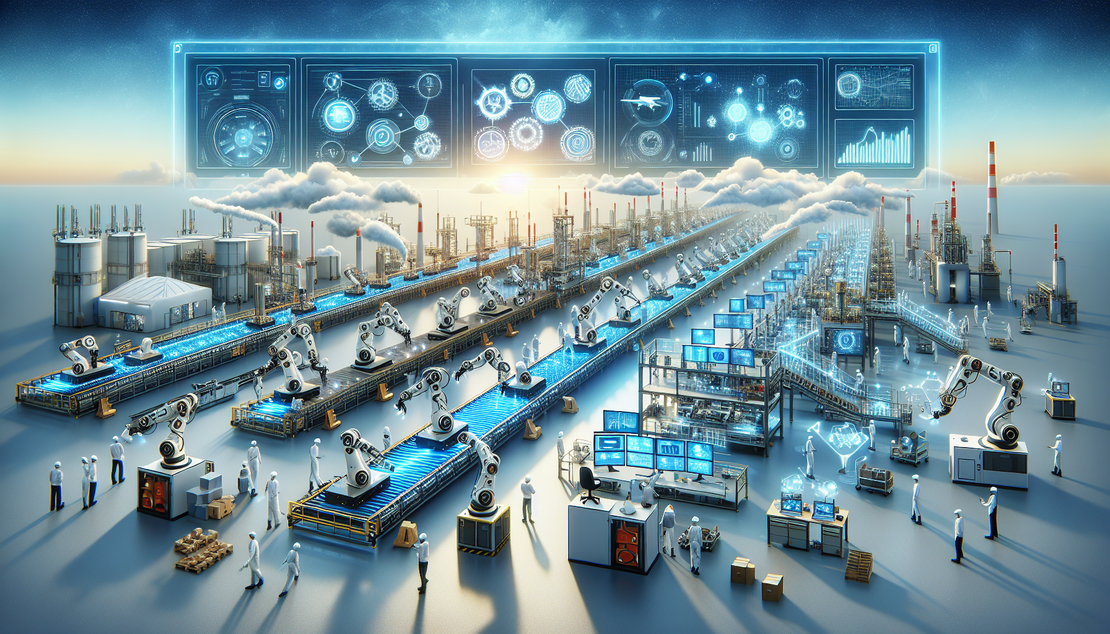
The future of Artificial Intelligence in Manufacturing: Optimizing Efficiency and Quality
- Rajdeep Barad
- Artificial intelligence in manufacturing , Ai automation , Optimizing efficiency , Machine learning , Manufacturing industry , Predictive maintenance
- December 25, 2024
The Future of Artificial Intelligence in Manufacturing: Optimizing Efficiency and Quality
Understanding the Impact of AI in Manufacturing
- Increased operational efficiency
- Improved product quality
- Enhanced predictive maintenance
- Optimized supply chain management
- Reduced downtime and production costs
Artificial Intelligence (AI) is revolutionizing the manufacturing industry by providing numerous benefits to businesses. Let’s delve into how AI impacts manufacturing processes:
Increased operational efficiency: AI streamlines operations by automating repetitive tasks, optimizing workflows, and reducing human error. This leads to enhanced productivity and operational efficiency.
Improved product quality: AI technologies like machine learning algorithms can analyze data in real-time to detect defects or anomalies, ensuring that products meet high-quality standards.
Enhanced predictive maintenance: AI can predict equipment failures before they occur, allowing for proactive maintenance scheduling, minimizing downtime, and extending the lifespan of machinery.
Optimized supply chain management: AI-driven systems analyze vast amounts of data to optimize inventory levels, predict demand fluctuations, and streamline logistics, leading to more efficient supply chains and reduced costs.
Reduced downtime and production costs: By predicting maintenance needs, optimizing processes, and improving quality control, AI helps reduce downtime, minimize production costs, and maximize overall profitability.
### Pro Tip: Implementing AI solutions in phased approaches allows for smoother integration and better adaptation by employees. Start with smaller, more manageable projects before scaling up to more complex AI systems.
AI Applications in Manufacturing
- Robotics and automation
- Predictive maintenance
- Quality control and defect detection
- Demand forecasting and inventory management
- Process optimization
AI applications in manufacturing cover a wide range of areas, enabling companies to operate more efficiently and competitively:
Robotics and automation: AI-powered robotics automate repetitive tasks, increasing production speed and accuracy while reducing labor costs.
Predictive maintenance: AI analyzes equipment data to predict maintenance needs, preventing unexpected breakdowns and reducing downtime.
Quality control and defect detection: AI algorithms can inspect products in real-time for defects, ensuring that only high-quality items reach the market.
Demand forecasting and inventory management: AI analyzes historical data and market trends to predict demand, optimize inventory levels, and minimize excess stock or shortages.
Process optimization: AI optimizes manufacturing processes by identifying inefficiencies, reducing waste, and improving overall resource utilization.
### Pro Tip: When implementing AI applications, ensure that the data collected is accurate and reliable to avoid biases or errors in the AI algorithms, leading to incorrect decisions.
The Role of Machine Learning in Manufacturing
- Training models for predictive maintenance
- Anomaly detection in production processes
- Optimizing production schedules
- Improving product design and customization
- Enhancing supply chain logistics with ML algorithms
Machine Learning (ML) plays a crucial role in transforming manufacturing processes and driving innovation. Here’s how ML benefits the manufacturing industry:
Training models for predictive maintenance: ML algorithms can analyze sensor data to predict equipment failure, enabling proactive maintenance and minimizing downtime.
Anomaly detection in production processes: ML can detect deviations from normal operations, such as equipment malfunctions or quality issues, ensuring consistent product quality.
Optimizing production schedules: ML algorithms optimize production schedules based on real-time data, demand forecasts, and resource availability, maximizing efficiency and minimizing delays.
Improving product design and customization: ML algorithms analyze customer preferences and feedback to customize products, improving customer satisfaction and brand loyalty.
Enhancing supply chain logistics with ML algorithms: ML algorithms optimize logistics by predicting delivery times, reducing transportation costs, and streamlining warehouse operations.
### Pro Tip: Regularly updating and retraining ML models with new data is essential to ensure their accuracy and effectiveness, especially as manufacturing processes evolve over time.
Challenges and Considerations in Implementing AI in Manufacturing
- Data security and privacy concerns
- Integration with existing systems
- Workforce upskilling and training
- Initial investment and ROI calculation
- Regulatory compliance and standards
While AI offers significant benefits to manufacturing, several challenges must be addressed to ensure successful implementation:
Data security and privacy concerns: Protecting sensitive manufacturing data from cyber threats and ensuring compliance with data privacy regulations is crucial when implementing AI systems.
Integration with existing systems: Integrating AI technologies with legacy systems and equipment can be complex and requires careful planning to ensure seamless operation and data exchange.
Workforce upskilling and training: Providing employees with the necessary skills and training to work alongside AI systems is essential for successful adoption and maximizing the benefits of AI in manufacturing.
Initial investment and ROI calculation: Determining the upfront costs of implementing AI systems, as well as the expected return on investment, is critical for decision-making and long-term success.
Regulatory compliance and standards: Ensuring that AI systems comply with industry regulations and standards is essential to avoid legal issues and maintain trust with customers and stakeholders.
### Pro Tip: Engaging with industry experts and seeking feedback from employees during the AI implementation process can help identify potential challenges early on and address them effectively.
Case Studies: Successful AI Implementations in Manufacturing
- Automating quality control at Toyota
- Using predictive maintenance at GE Aviation
- Optimizing production with machine learning at Foxconn
- Improving inventory management at Siemens
- Enhancing design processes at Airbus
Real-world examples of AI implementations in manufacturing showcase the tangible benefits and successes that companies have achieved:
Automating quality control at Toyota: Toyota uses AI-powered robotics and image recognition for quality control, ensuring consistent product quality and reducing defects.
Using predictive maintenance at GE Aviation: GE Aviation utilizes AI algorithms to analyze sensor data and predict maintenance needs, improving aircraft reliability and reducing downtime.
Optimizing production with machine learning at Foxconn: Foxconn leverages machine learning algorithms to optimize production schedules, reduce lead times, and enhance efficiency in manufacturing operations.
Improving inventory management at Siemens: Siemens uses AI for demand forecasting and inventory optimization, leading to reduced carrying costs, lower stockouts, and improved customer service.
Enhancing design processes at Airbus: Airbus integrates AI in its design processes to streamline product development, improve customization options, and enhance overall innovation in aircraft design.
### Pro Tip: Studying successful AI case studies in manufacturing can provide valuable insights and inspiration for implementing AI solutions in your own production processes, guiding you towards improved efficiency and quality.
Ready to transform your digital vision?
Get in touch with us to explore how our cutting-edge solutions can elevate your business to new heights. Contact us today!
Get in Touch